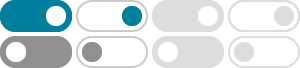
Audio Super-Resolution With Robust Speech Representation …
Recently, masked autoencoders have been found to be beneficial in learning robust representations of audio for speech classification tasks. Following these studies, we leverage these representations and investigate several masking strategies for …
facebookresearch/AudioMAE - GitHub
This repo hosts the code and models of "Masked Autoencoders that Listen". Resources
A deep learning framework for audio restoration using …
Nov 15, 2023 · A bubble chart has been provided in Fig. 6 to show which combination of use case and audio length has a greater number of equal values when comparing their waveforms. As can be seen, the use case of audio with noise works better in this case.
sh-lee-prml/sh-lee-prml - GitHub
Audio Super-resolution with Robust Speech Representation Learning of Masked Autoencoder, S.-B. Kim, S.-H. Lee, H.-Y. Choi, S.-W. Lee, IEEE Trans. on Audio, Speech and Language Processing, 2024.
Our work also uses the masked autoencod-ing framework, but jointly models both audio and video, and is demonstrated on both unimodal (i.e. video-only and audio-only) and audiovisual downstream tasks where it out-performs supervised pretraining.
(PDF) Audio Super-Resolution With Robust Speech
Jan 1, 2024 · In this paper, we propose an upper-band masking strategy with the initialization of the mask token, which is simple but efficient for audio super-resolution. Furthermore, we propose a...
Overall framework of Fre-Painter. Initially, we pre-train the masked ...
Initially, we pre-train the masked autoencoder using a random masking strategy. Subsequently, the generator is jointly trained with the pre-trained encoder of masked autoencoder. For audio...
AudioSR: Versatile Audio Super-resolution at Scale - GitHub Pages
We introduce a diffusion-based generative model, AudioSR, that is capable of performing robust audio super-resolution on versatile audio types, including sound effects, music, and speech. Specifically, AudioSR can upsample any input audio signal within the bandwidth range of 2 kHz to 16 kHz to a high-resolution audio signal at 24 kHz bandwidth ...
In this paper, we propose Fre-Painter, a robust neural audio super-resolution system that utilizes robust speech represen-tation learning using MAE and several masking strategies. We utilize a...
[2207.06405] Masked Autoencoders that Listen - arXiv.org
Jul 13, 2022 · This paper studies a simple extension of image-based Masked Autoencoders (MAE) to self-supervised representation learning from audio spectrograms. Following the Transformer encoder-decoder design in MAE, our Audio-MAE first encodes audio spectrogram patches with a high masking ratio, feeding only the non-masked tokens through encoder layers.
- Some results have been removed