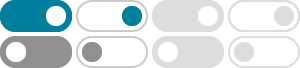
Masked Autoencoders in 3D Point Cloud Representation Learning
Jul 4, 2022 · In this paper, we propose masked Autoencoders in 3D point cloud representation learning (abbreviated as MAE3D), a novel autoencoding paradigm for self-supervised learning. We first split the input point cloud into patches and mask a portion of them, then use our Patch Embedding Module to extract the features of unmasked patches.
GitHub - Pang-Yatian/Point-MAE: [ECCV2022] Masked …
In this work, we present a novel scheme of masked autoencoders for point cloud self-supervised learning, termed as Point-MAE. Our Point-MAE is neat and efficient, with minimal modifications based on the properties of the point cloud.
Masked Autoencoder for Pre-Training on 3D Point Cloud Object …
Sep 28, 2022 · This paper proposes Point Cloud Masked Autoencoder (PCMAE), which can provide pre-training for most voxel-based point cloud object detection algorithms. PCMAE improves the feature representation ability of the 3D backbone for long-distance and occluded objects through self-supervised learning.
3D Feature Prediction for Masked-AutoEncoder-Based Point Cloud ...
Jan 16, 2024 · Masked autoencoders (MAE) have recently been introduced to 3D self-supervised pretraining for point clouds due to their great success in NLP and computer vision. Unlike MAEs used in the image domain, where the pretext task is to restore features at the masked pixels, such as colors, the existing 3D MAE works reconstruct the missing geometry ...
Rethinking Masked-Autoencoder-Based 3D Point Cloud …
To address these issues, we propose LSV-MAE, a masked autoencoding pre-training scheme designed for voxel representations. We pre-train the backbone to reconstruct the masked voxels features extracted by PointNN.
Masked Autoencoders for 3D Point Cloud Self-supervised Learning
In this paper, we propose a novel scheme of masked autoencoders for 3D point cloud self-supervised learning, addressing the special challenges posed by point cloud, including leakage of location information and uneven information density.
Masked Autoencoders for Point Cloud Self-supervised Learning
Mar 13, 2022 · Inspired by this, we propose a neat scheme of masked autoencoders for point cloud self-supervised learning, addressing the challenges posed by point cloud's properties, including leakage of location information and uneven information density.
To address the fusion of multi-modal point cloud and im-age data, we propose PiMAE, a simple yet effective pipeline that learns strong 3D and 2D features by increasing their interaction.
Self-Supervised Learning for 3-D Point Clouds Based on a Masked …
Motivated by the success of a masked autoencoder in 3-D point-cloud-based learning, this study proposes an innovative framework for self-supervised learning (SSL) on 3-D point clouds with linear complexity. In the proposed framework, every input point cloud is divided into multiple point patches, which are randomly masked at different ratios.
Evaluating masked self-supervised learning frameworks for 3D …
4 days ago · Yu, X. et al. Point-Bert: Pre-training 3d point cloud transformers with masked point modeling. In Proceedings of the IEEE/CVF Conference on Computer Vision and Pattern Recognition 19313–19322 ...